Onboard more customers and avoid regulatory fines
Easily configure your KYC workflow with a layered approach, applying more friction for risky customers while showing trusted ones the fast lane.
Detect financial crime in real-time with AI
Detect and block fraudsters early with real-time detection bolstered by machine learning and investigative tools to analyze suspicious transactions.
Automate to boost efficiency and accuracy
Reduce the drain on resources with automation across the AML compliance life cycle, streamlined investigations and comprehensive reporting.
Building trusted customer relationships for businesses worldwide
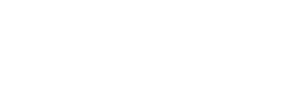
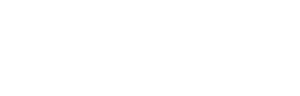
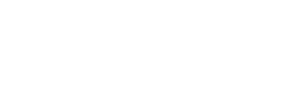
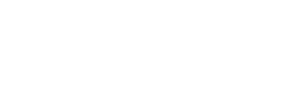
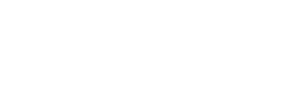
Manage risk and stop fraudsters in their tracks
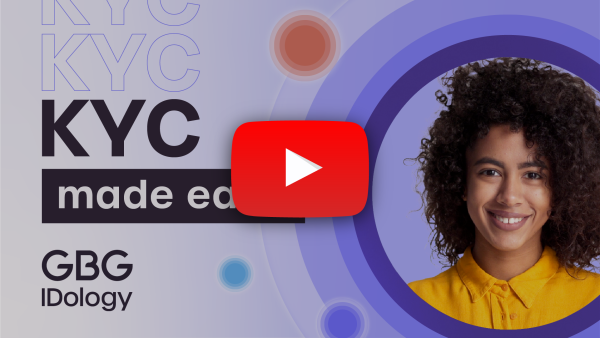
Complying with anti-money laundering (AML) and know your customer (KYC) regulations can be costly, complex and, if not done right, can derail growth. Our award-winning identity verification and fraud prevention solutions deliver complete regulatory protection and enable you to build a good reputation as an organization that takes care of its customers and clients.
Comprehensive AML solutions address the full compliance life cycle, with Know Your Customer (KYC) and Know Your Business (KYB) capabilities, risk ratings, continuous PEPs and sanctions screening and transaction monitoring. Alerts and case management tools help analysts investigate financial crimes and quickly resolve exceptions.
No matter what industry your business operates in, our solutions can be easily integrated into your existing processes, allowing for additional protection against fraud, full KYC and AML compliance and a superior customer experience.
Key benefits
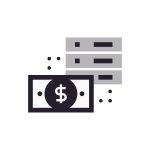
Easily configure your solution and workflow
Our KYC/AML solutions can be flexibly configured to your business needs and industry requirements, supporting extensive risk profiles, which can be built to automatically accept, decline, and refer decisions.
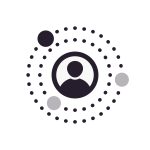
Monitor risk continuously and protect your brand
Routinely monitor identities, reputations, and transactions to detect and manage risk over time— safeguarding your customers and your reputation.
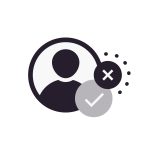
Ensure you reduce false positives
Reduce the flood of false positives and irrelevant alerts that plague compliance teams using machine learning to prioritize critical alerts.
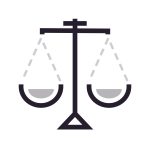
Rapidly adapt to changing regulations
Adapt to changing standards and compliance needs in the markets you service, and easily meet industry regulations when entering new markets globally.
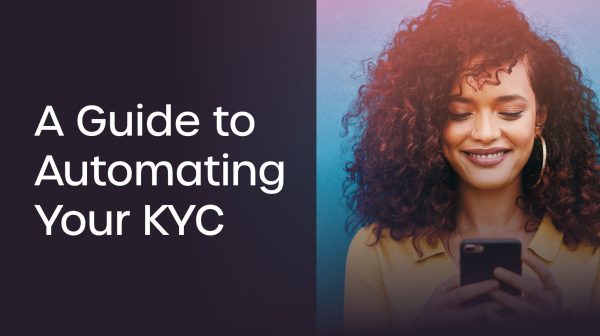
Guide: Automating your KYC
Automated KYC is imperative for businesses to stay competitive today. This guide will help you make informed decisions when choosing an end-to-end KYC compliance platform without making compromises. Ensure AML compliance, improve your operational efficiency and provide a superior customer experience.